Introduction
Effective data management is crucial in the realm of clinical trials, where the integrity and accessibility of data directly impact the veracity of outcomes. From cross-border trials to standardized frameworks for assessing data relevance and reliability, this article explores the importance of data management in clinical trials.
It delves into the challenges, key elements, processes, and best practices for managing clinical trials data. Additionally, it examines the role of data management in ensuring data quality, the tools and technologies used in clinical data management, regulatory compliance, and future directions in data management. Join us as we delve into the intricacies of effective data management in clinical trials.
Importance of Effective Data Management in Clinical Trials
In the intricate web of clinical trials, data management emerges as a linchpin, ensuring the veracity and dependability of the outcomes. For instance, consider the plight of a patient in rural Pennsylvania grappling with an ultra-rare disease. Offered a lifeline through a clinical trial in Turkey, the patient faces daunting logistical hurdles, from visa procurement to navigating foreign documentation.
The integrity and accessibility of data become paramount in such cross-border trials, where the transfer of records and related data between vendors and sponsors necessitates meticulous mapping. As highlighted by Flatiron's recent publication, which garnered attention from FDA members, the quality of real-world data is pivotal. The research underscores the necessity for a standardized framework to assess data relevance and reliability in informing scientific deductions.
Clinical trials, pivotal in shaping patient care, are meticulously structured into phases, each with stringent goals to ascertain the safety and efficacy of new interventions. Phase one trials, with a small cohort of healthy volunteers, prioritize safety, while phase two expands to include patients, gauging the treatment's efficacy. Robust data management systems are thus not just beneficial but crucial in upholding the standards of clinical trials, ensuring that each phase yields results that are both trustworthy and actionable.
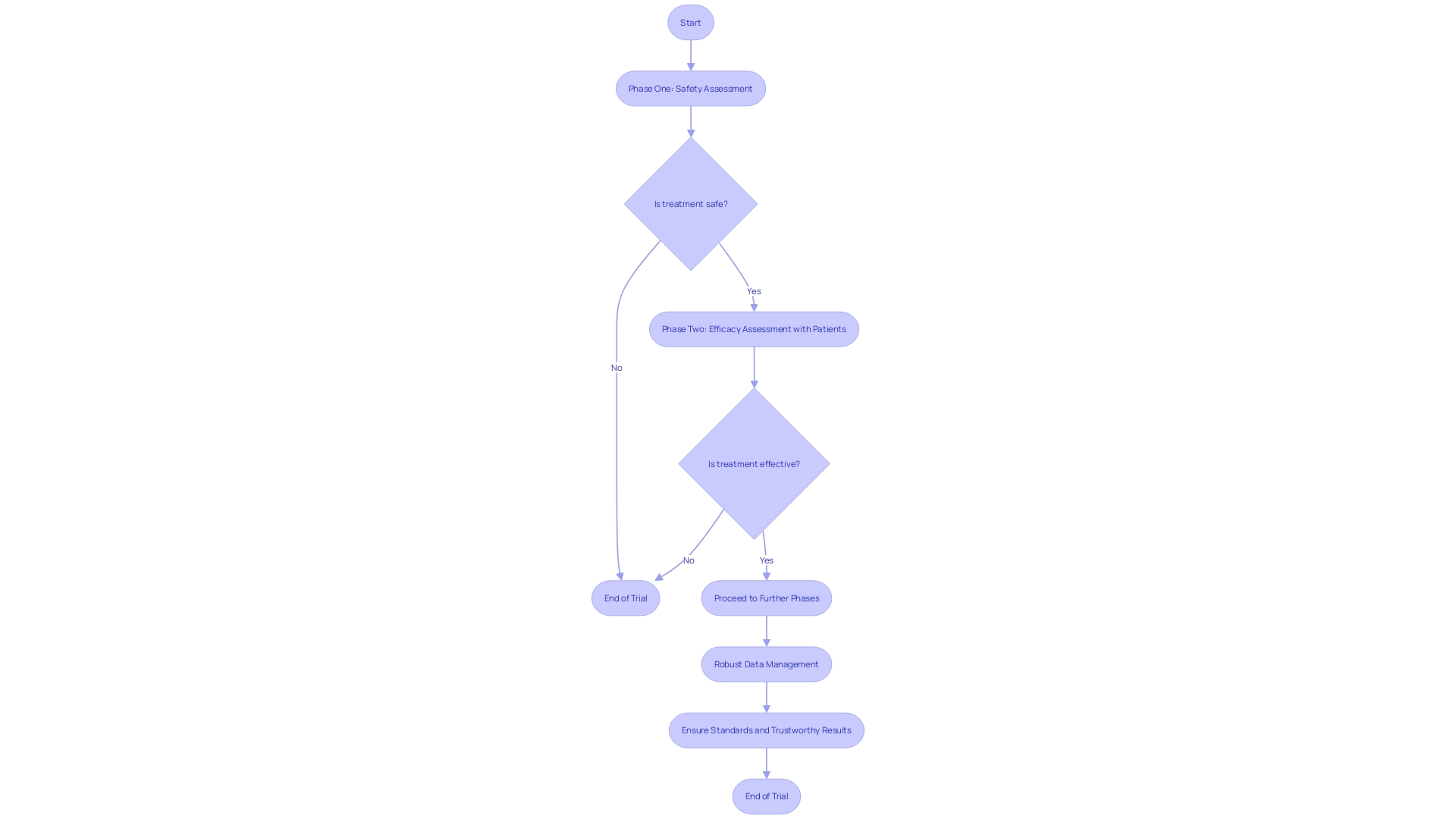
Challenges in Clinical Trials Data Management
The landscape of clinical trials is fraught with complexities, and managing the myriad of data they generate is a task that demands precision and strategic planning. Consider the plight of a patient from rural Pennsylvania with an ultra-rare disease, who is offered participation in a clinical trial based in Turkey.
This scenario underscores the logistical hurdles and data management intricacies involved when dealing with international trials. Not only must patients navigate personal challenges such as visa procurement and language barriers, but researchers must also ensure the seamless integration and quality of data across borders.
The stakes are high in clinical research, where the balance between innovation and patient safety is delicate. As artificial intelligence (AI) and machine learning (ML) technologies gain traction, regulatory bodies are intensifying scrutiny to mitigate risks.
The EU AI Act, for instance, advocates a 'risk-based approach' to AI in healthcare, demanding transparency and adherence to stringent guidelines. Such regulatory vigilance is a testament to the industry's commitment to data integrity. After all, in an ideal world where high-quality data is paramount, systematic reviews and meta-analyses would be the cornerstones of medical decision-making. Yet, the variability inherent in human subjects and the effects of medical interventions make drawing accurate conclusions challenging. In light of these hurdles, the clinical research community must be unwavering in its pursuit of data excellence to ensure the safety and efficacy of treatments, be they drugs, devices, or biologics.
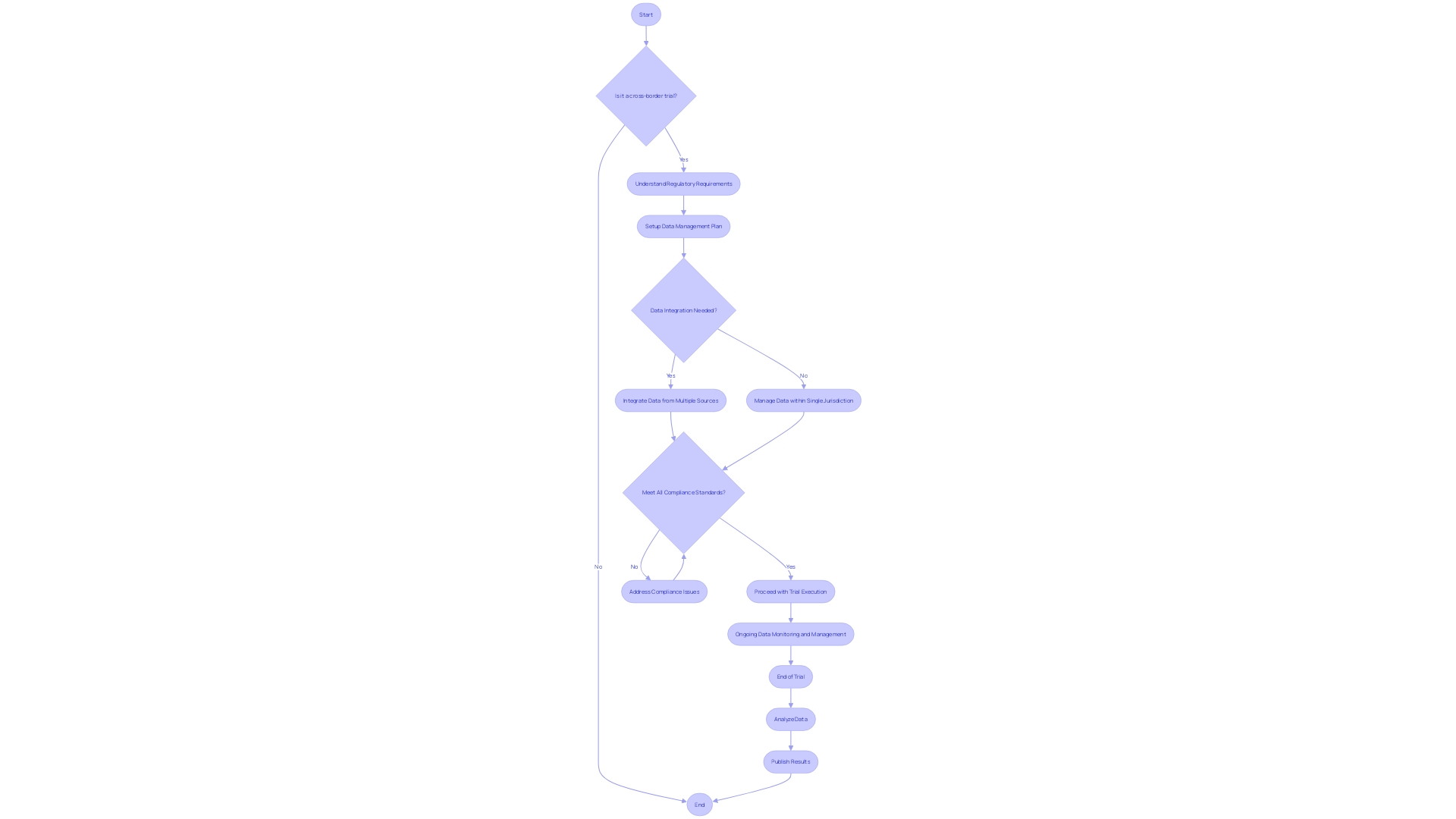
Key Elements of Clinical Trials Data Management
In the realm of medical research, specifically within clinical trials, the management of data is a paramount concern. The matrix structure is recommended for the collection of medical research data, where each row represents a single observation, typically a research participant, and columns represent participant characteristics, or variables.
This systematic approach ensures data integrity and facilitates subsequent analysis and reporting. The importance of this approach is underscored by statistics indicating that data preparation, which includes the process of cleaning data for use in models, consumes about 80% of a data scientist's time, according to Forbes.
The mean time to production (MTTP), which reflects the average time to market for data products, can be directly affected by the quality of data management practices. Moreover, the mean time to resolution (MTTR) for data incidents is contingent upon the complexity of the data platform and the incident's severity. Clinical trials, which are critical for establishing the safety and efficacy of new treatments ranging from drugs to devices, must adhere to these methodical data management practices to maintain the high standards required for patient care outcomes. These trials span several phases, with phase one focusing on safety in a small group of healthy volunteers, phase two expanding to assess efficacy and safety in a larger participant group, including those with the targeted condition, and further phases to confirm effectiveness and monitor side effects.
Data Management Processes in Clinical Trials
In the realm of clinical trials, the management of data is a critical component that underpins the entire research process. These trials are pivotal in testing and establishing the safety and efficacy of new medical interventions—ranging from pharmaceuticals to behavioral strategies, and innovative medical devices—ultimately determining their suitability for patient application.
Not only do clinical trials have the potential to unveil more effective treatment options, but they also serve to identify treatments with reduced adverse effects compared to existing alternatives. To ensure the credibility of trial outcomes, which have a direct impact on patient care, a meticulous approach to data management is indispensable.
It begins with the careful design of data collection methodologies and the selection of precise tools, followed by the formulation of exacting standards for data entry. Vigilant data quality assessments are carried out routinely, accompanied by thorough data cleansing initiatives to uphold the integrity of the data. Secure data storage solutions are employed to protect the information gathered, and robust data analysis methods are utilized to validate the findings. The diligent adherence to these data management steps is essential for preserving the integrity of the data and confirming that the results of the trial are both reliable and in compliance with regulatory standards.
Role of Clinical Data Management in Ensuring Data Quality
In the landscape of clinical research, the management of data is not just a supplementary task; it is a cornerstone of trial integrity. The healthcare industry, which now represents approximately 30% of the world's data volume and is expected to grow annually by 36% by 2025, is increasingly reliant on high-quality data.
Within this context, standardized data collection protocols are essential in mitigating errors and ensuring consistency. The process of data validation and cleaning is critical to rectify inaccuracies or gaps, thereby solidifying the foundation upon which clinical decisions are made.
Rigorous quality checks and audits are not mere formalities; they are pivotal in enhancing the trustworthiness of data. As the volume and significance of healthcare data surge, underpinned by its use in medical research, pharmaceuticals, AI algorithm refinement, and healthcare operations, the importance of meticulous data management grows exponentially. It not only supports the development of data-led healthcare offerings but also propels innovation, driving forward advancements in drug discovery and medtech, and ultimately contributing to enhanced patient care.
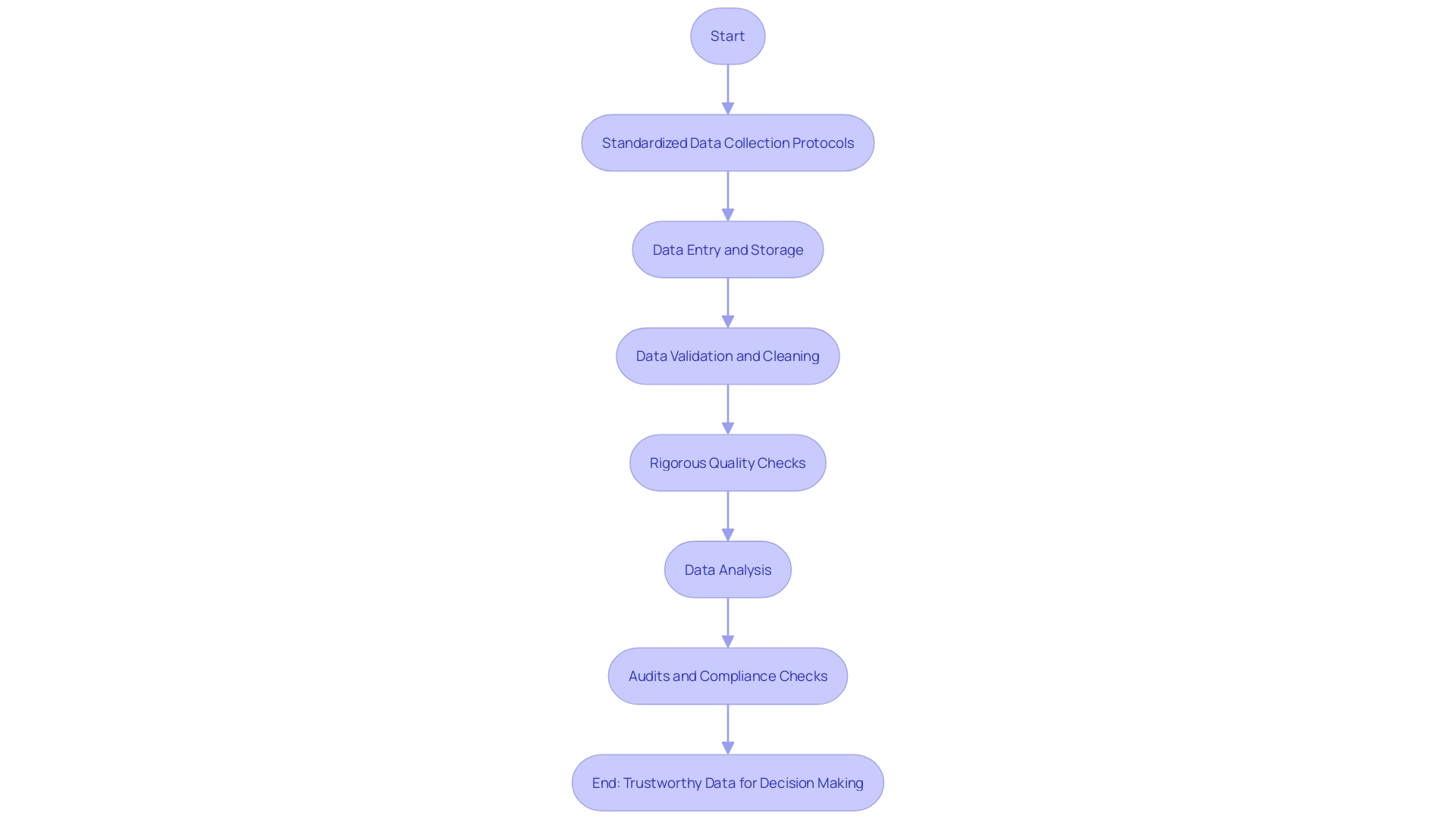
Best Practices for Clinical Trials Data Management
In the realm of clinical trials, the collection and management of data are paramount to the success and integrity of the research. A poignant example is seen in the case of a patient from rural Pennsylvania with an ultra-rare disease.
Faced with no FDA-approved treatments locally, they are presented with the chance to join a clinical trial abroad in Turkey. Here, the complexities of data management become evident, as the patient grapples with the logistics of international travel, language barriers in document handling, and the intricacies of cross-border regulations.
To navigate such challenges, adherence to a set of clear and concise rules is essential. As per the guidance of experts in the field, data should be meticulously organized in a matrix format, where each row represents an individual observation—typically a patient—and the columns denote the variables pertaining to those participants.
Moreover, the importance of understanding and following regulations cannot be overstated. Privacy, security, and accountability must be woven into the fabric of data science practices. Familiarity with the relevant rules and laws, and the ability to apply them, is not just about compliance—it’s about embracing a culture of codified best practices that can vastly improve the quality of data management. Implementing standardized data collection forms, training personnel thoroughly, ensuring regular quality checks, embracing electronic data capture for efficiency, securing data storage and transmission, and keeping detailed documentation are the pillars of obtaining reliable data. Following these steps, researchers can ensure that data is collected and managed effectively, minimizing errors and facilitating the analysis and reporting of results, which is crucial for the advancement of medical science and patient care.
Tools and Technologies for Clinical Data Management
In the realm of healthcare, effective data management is paramount for delivering quality care and maintaining operational efficiency. With hospitals generating an immense 50 petabytes of data annually, it becomes critical to harness this information to its full potential. Yet, a staggering 97% of this data remains untapped, representing a significant loss in opportunities.
The integration of disparate healthcare systems through mergers and acquisitions has laid a stronger foundation for medical services but simultaneously has introduced complex data management challenges. IT teams are tasked with creating scalable solutions to handle data from various source systems, often leading to increased administrative costs and reduced employee productivity. To mitigate these challenges, Electronic Data Capture (EDC) systems are instrumental.
They not only facilitate efficient and secure data entry but also ensure that the storage and analysis of data are compliant and accurate. These systems are equipped with data validation checks and automated cleaning algorithms to maintain data integrity. Moreover, they provide customizable reporting capabilities, enabling healthcare organizations to make informed decisions.
As the industry evolves, it's essential to embrace a shift in mindset regarding data strategy, investing in modern tools that offer 'pay as you go' models. This pragmatic approach, as experts suggest, can significantly alter the cost dynamics, allowing health organizations to optimize their expenditure and focus on patient outcomes. Embracing data portability can bridge the gaps in patient health records, aggregating data from diverse systems to provide a more comprehensive view of patient health, despite the absence of a complete whole-person record.
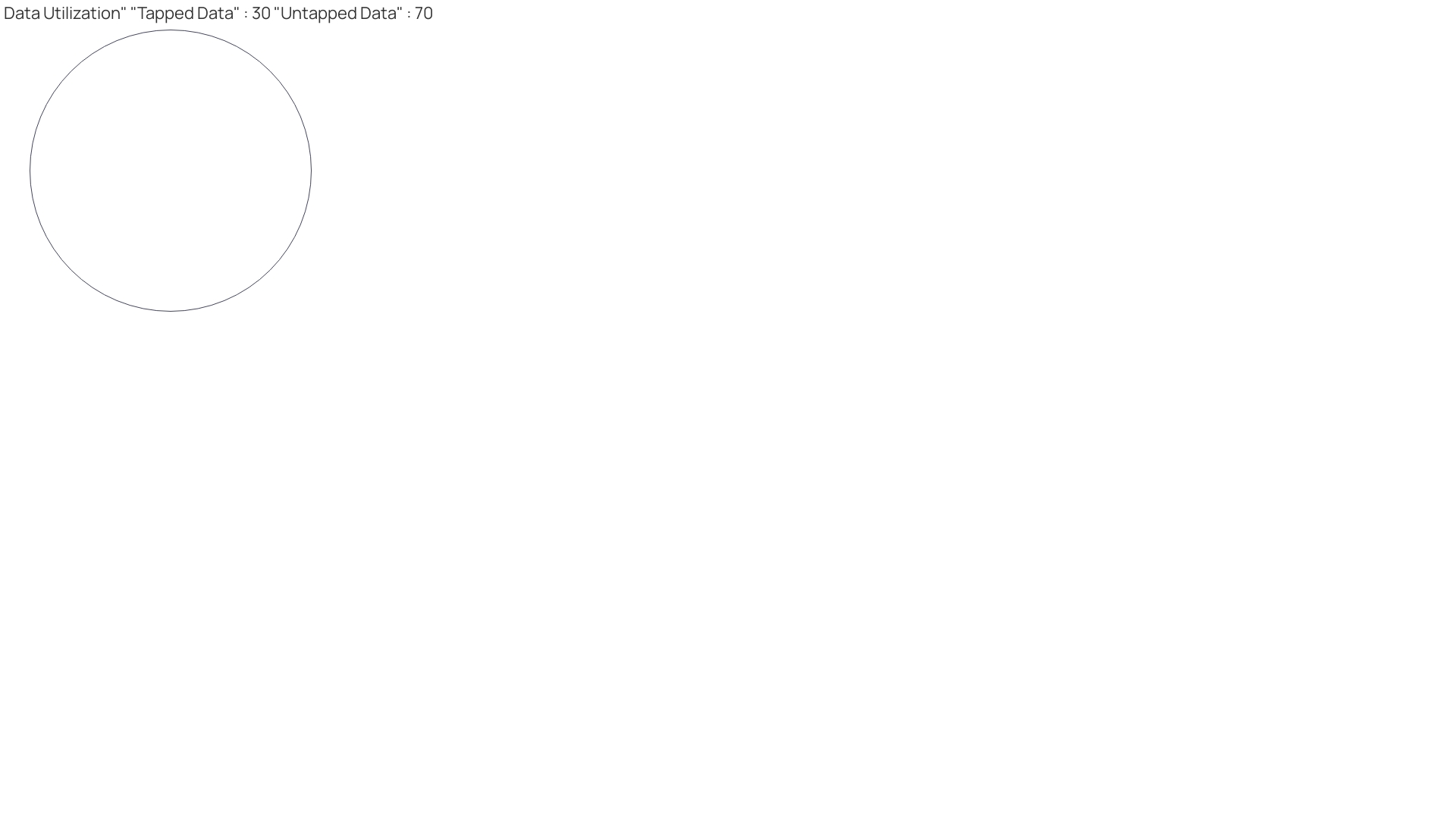
Regulatory Compliance in Clinical Trials Data Management
Navigating the complex landscape of clinical trials requires a delicate balance between embracing innovative technologies like artificial intelligence (AI) and machine learning (ML), and maintaining patient safety through stringent regulatory compliance. New developments in technology are propelling the healthcare industry forward, yet they also bring heightened risks that regulatory bodies such as the FDA, EU, and EMA are diligently working to mitigate. These organizations are crafting guidelines, like the EU AI Act, that advocate for a risk-based approach to AI usage and demand transparency from technology providers.
Clinical trials play a pivotal role in medical research, testing new treatments and interventions to establish their safety and effectiveness for patient use. Each of the three main stages of clinical trials—starting with a small cohort of healthy volunteers in phase one to assess safety, moving to larger groups including patients in phase two to evaluate both efficacy and safety—must adhere to rigorous and well-defined standards. This ensures that any new treatment is not only safe but also potentially more effective or with fewer side effects than existing options, directly impacting patient care and outcomes.
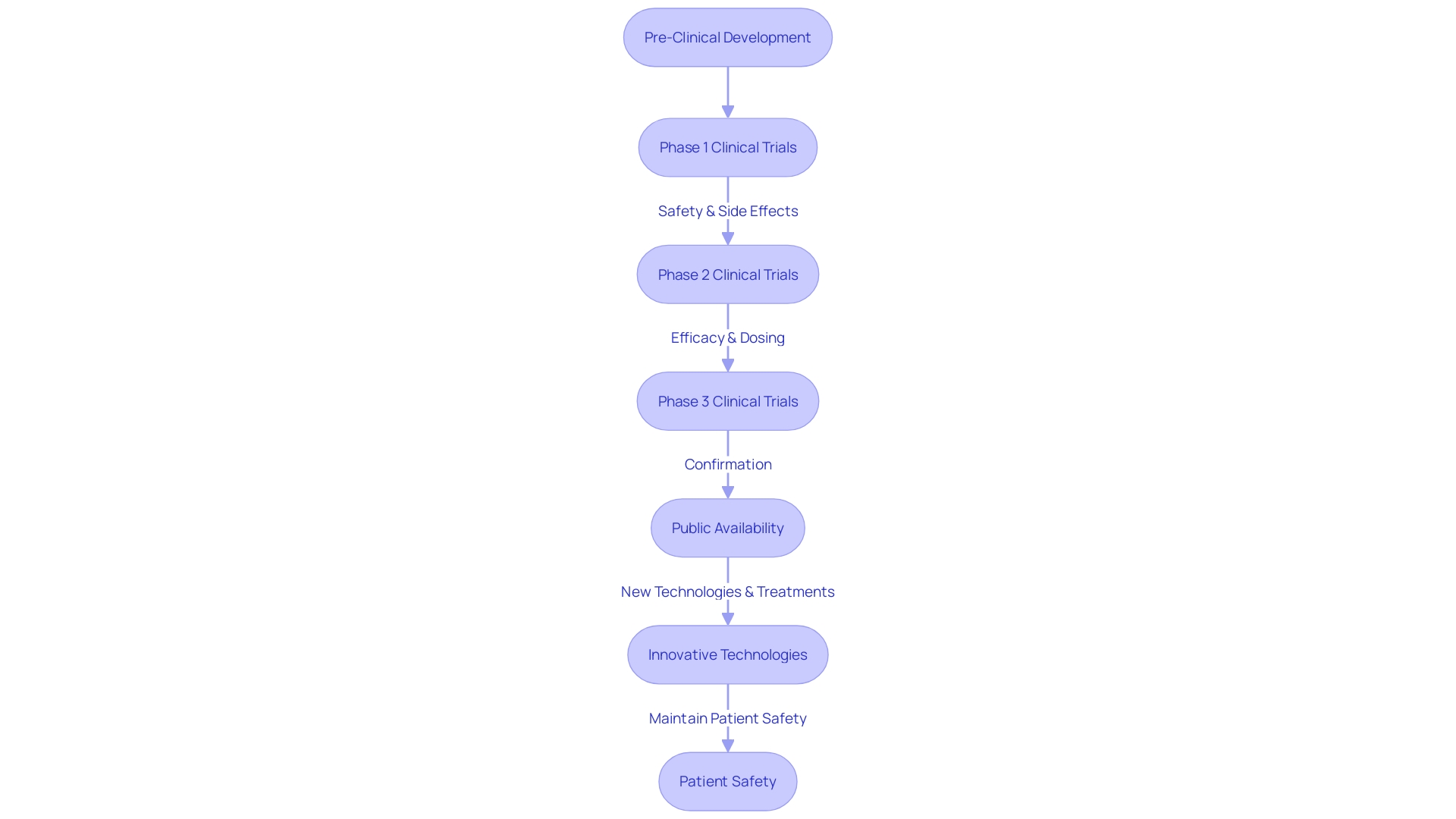
Future Directions in Clinical Trials Data Management
As the landscape of clinical trials data management rapidly transforms, the integration of cutting-edge technologies is pivotal in enhancing the process. The surge of data within the healthcare sector is staggering, with medical information now doubling every 70 days compared to every 50 years in 1950. A contemporary Phase 3 trial amasses an average of 3.6 million data points, a threefold increase from the volume recorded a decade ago.
The advent of artificial intelligence (AI) and machine learning (ML) algorithms is set to revolutionize data management by automating intricate tasks, elevating data precision, and enabling instantaneous data monitoring. Concurrently, blockchain technology emerges as a potential game-changer for its ability to fortify data storage and sharing, bolstering data integrity and transparency. These advancements underscore the necessity for researchers to remain abreast of technological progress and to adeptly integrate novel methodologies to boost the efficiency and effectiveness of managing clinical trials data.
Conclusion
In conclusion, effective data management is crucial in clinical trials. It ensures the integrity and accessibility of data, especially in cross-border trials. Standardized frameworks for assessing data relevance and reliability are essential.
Challenges in clinical trials data management include logistical hurdles and maintaining a balance between innovation and patient safety. Regulatory bodies are intensifying scrutiny to ensure data integrity. Key elements of data management involve systematic collection, ensuring integrity, and facilitating analysis.
Adherence to these steps maintains the integrity of trial results. Data management processes include careful design, precise tools selection, quality assessments, thorough cleansing initiatives, secure storage solutions, and robust analysis methods. Clinical data management ensures quality through standardized protocols, validation, cleaning, quality checks, audits, secure storage solutions, and robust analysis methods.
Best practices include organizing data in a matrix format, following regulations for privacy and security, implementing standardized forms, training personnel thoroughly, conducting regular quality checks, embracing electronic data capture for efficiency, securing storage and transmission of data. Tools like Electronic Data Capture (EDC) systems facilitate efficient and secure entry while ensuring compliance and accuracy. They provide customizable reporting capabilities.
Regulatory compliance is essential in navigating the complex landscape of clinical trials. Rigorous standards must be adhered to at each stage to establish safety and effectiveness. Future directions involve integrating AI algorithms for automation and real-time monitoring.
Blockchain technology holds promise for fortifying storage and sharing while boosting integrity. In summary, effective data management is pivotal in ensuring credible trial outcomes that impact patient care. By following best practices and utilizing appropriate tools responsibly, researchers can optimize their efforts in managing valuable clinical trials' data.